Chemical Constituents, Insecticidal Activity, Herbicidal Potential and Soft Computing Analysis in Foeniculum vulgare Mill. (Fennel): A Bioherbicide
DOI:
https://doi.org/10.29356/jmcs.v69i2.2075Keywords:
ANN, bioherbicide, Foeniculum vulgare, fuzzy-c-means clustering, insecticidal activityAbstract
Abstract. To develop an environment friendly natural herbicide is a new topic of interest in the agricultural era over the worldwide. Nowadays people are more concerned about their health in consideration to nutrition related problems for the better quality of life. The aim of present study is to examine the phytoconstituents and biological efficacy in the fennel seed collected from Tarai region in the Uttarakhand state. Chemical constituents, insecticidal activity and herbicidal potential in hexane, chloroform and methanol extract of fennel seed were evaluated. Soft computing analysis was done in terms of fuzzy-c-means clustering (FCM) and artificial neural network (ANN). FNL-120, FNL-125 and FNL-PM exhibits highest mortality rate in methanol and hexane extract of fennel against Tribolium castaneum and S. oryzae. Herbicidal activity in hexane, chloroform and methanol extract of fennel seed was analyzed by observing the seed germination inhibition potential in radish (Raphanus sativus) seeds. Methanol extract of FNL-PM have effective seed germination inhibition activity with IC50 =549.10 ±2.86 μg/ mL. Due to significant complexity of herbicidal activity (IC50 value) of fennel, having different concentrations of extract, traditional approaches to biological study struggle to provide meaningful outcomes. To rectify, the simulation data are intensify into an artificial neural network to evaluate the target responses. Fennel extract have higher seed germination inhibition potential can be used as a natural herbicide to control weed growth for the better yield of crop and a good initiative to develop sustainable management of weed.
Resumen. Desarrollar un herbicida natural respetuoso con el medio ambiente es un tema de interés en la era agrícola mundial. Hoy en día, las personas se preocupan más por su salud que por los problemas nutricionales para una mejor calidad de vida. El objetivo del presente estudio es examinar los fitoconstituyentes y la eficacia biológica de las semillas de hinojo recolectadas en la región de Tarai, estado de Uttarakhand. Se evaluaron los componentes químicos, la actividad insecticida y el potencial herbicida de los extractos de hexano, cloroformo y metanólico de las semillas de hinojo. Se realizó un análisis computacional suave mediante agrupamiento difuso de c-medias (FCM) y redes neuronales artificiales (ANN). FNL-120, FNL-125 y FNL-PM presentaron la mayor tasa de mortalidad en extractos de hinojo con metanol y hexano contra Tribolium castaneum y S. oryzae. Se analizó la actividad herbicida de los extractos de hexano, cloroformo y metanólico de las semillas de hinojo observando el potencial de inhibición de la germinación de las semillas de rábano (Raphanus sativus). El extracto metanólico de FNL-PM presenta una actividad inhibidora eficaz de la germinación de las semillas con una CI50 de 549,10 ±2,86 μg/mL. Debido a la considerable complejidad de la actividad herbicida (CI50) del hinojo, con diferentes concentraciones de extracto, los enfoques tradicionales de estudios biológicos presentan dificultades para obtener resultados significativos. Para solucionar este problema, los datos de simulación se intensifican en una red neuronal artificial (RNA) para evaluar las respuestas objetivo. El extracto de hinojo, con un mayor potencial de inhibición de la germinación de las semillas, puede utilizarse como herbicida natural para controlar el crecimiento de malezas, lo que mejora el rendimiento del cultivo y constituye una buena iniciativa para el desarrollo de la gestión sostenible de malezas.
Downloads
References
Goswami, N.; Chatterjee, S. Biomed Res. Int. 2014. DOI: https://doi.org/10.1155/2014/582767.
Olaleye, M.T. J. Med. Plants Res. 2007, 1, 9–13.
Sabzi Nojadeh, M.; Pouresmaeil, M.; Younessi-Hamzekhanlu, M.; Venditti, A. Nat. Prod. Res. 2021, 35, 4164-4168. DOI: https://doi.org/10.1080/14786419.2020.1741580.
Mehra, N.; Tamta, G.; Nand, V.; Singh, J. P. J. Food Process. Preserv. 2022, 46, e16763. DOI: https://doi.org/10.1111/jfpp.16763.
Hamid, A. A.; Aiyelaagbe, O. O.; Usman, L. A.; Ameen, O. M.; Lawal, A. Afr. J. Pure Appl. Chem. 2010, 4, 142-151.
Dorra, N.; El-Berrawy, M.; Sallam, S.; Mahmoud, R. J. High Inst. Public Health. 2019, 49, 36-40. DOI: 10.21608/JHIPH.2019.29464.
Mehra, N.; Tamta, G.; Nand, V. J. Pharmacog Phytochem. 2021, 10, 1255-1263.
Grover, S.; Malik, C. P.; Hora, A.; Kushwaha, H. B. Int. J. Life Sci. 2013, 28-139.
Khandelwal, K. R. Preliminary phytochemicals screening: Practical Pharmacognosy. 2001,149-156.
Castaldo, L.; Izzo, L.; De Pascale, S.; Narváez, A.; Rodriguez-Carrasco, Y.; Ritieni, A. Molecules. 2021, 26, 1968. DOI: https://doi.org/10.3390/molecules26071968.
Bahmani, K.; Darbandi, A. I.; Ramshini, H. A.; Moradi, N.; Akbari, A. Ind. Crops Prod. 2015, 77, 82-94. DOI: https://doi.org/10.1016/j.indcrop.2015.08.059.
Mehra, N.; Tamta, G.; Nand, V. Ind. J. Nat. Prod. Res. 2022, 13, 213-222. DOI: 10.56042/ijnpr.v13i2.51347.
Digilio, M. C.; Mancini, E.; Voto, E.; De Feo, V. J. Plant Interact. 2008, 3, 17-23. DOI: https://doi.org/10.1080/17429140701843741.
Regnault-Roger, C. Integ. Pest Management Rev. 1997, 2, 25-34.
Zerroug, E.; Belaidi. S.; Chtita, S. J. Chin. Chem. Soc. 2021, 68, 1379–99. DOI: https://doi.org/10.1002/jccs.202000457.
Garkani-Nejad, Z.; Saneie, F. Bull. Chem. Soc. Ethiop. 2010, 24. DOI: 10.4314/bcse.v24i3.60661.
Abdullah, H.; Chia, P.W.; Omar, D.; Chuah, T.S. Sci Rep. 2021, 11, 1–13.
Erenler. R.; Demirtas, I.; Karan, T.; Gul, F.; Kayir, O.; Karakoc, O. C. Trends in Phytochem. Res. 2018, 2, 91-6.
Sahu, A.; Devkota, A. Sci. World J. 2013, 11, 90-93.
Bezdek, J. C. Pattern Recognition with Fuzzy Objective Function Algorithms. 1981, 1-3. DOI https://doi.org/10.1007/978-1-4757-0450-1.
MathWorks - Makers of MATLAB and Simulink - MATLAB & Simulink. https://www.mathworks.com/.
Agrwal, A.; Verma, A.; Chantola, N.; Verma, S.; Kasana, V. J Environ. Sci. Health B. 2022, 57, 379-420. DOI: https://doi.org/10.1080/03601234.2022.2062188.
Mallik, S.; Sharangi, A. B.; Sarkar, T. Nat. Acad. Sci Lett. 2022, 43, 477-80. DOI: https://doi.org/10.1007/s40009-020-00884-5.
Bano, S.; Ahmad, N.; Sharma, A. K. Int. J. Pharma Sci Res. 2016, 7, 310-4.
Mehra, N.; Garima, T.; Nand, V. Ind. J. Nat. Prod. Res. 2022, 13, 213-222. DOI: https://doi.org/10.56042/ijnpr.v13i2.51347.
Mehra, N.; Garima, T.; Nand, V. Ind. J. Nat. Prod. Res. 2023, 14, 372-383. DOI: https://doi.org/10.56042/ijnpr.v14i3.4598.
Pavela, R.; Žabka, M.; Bednář, J.; Tříska, J.; Vrchotová, N. Ind. Crops. Prod. 2016, 1, 275-82. DOI: https://doi.org/10.1016/j.indcrop.2015.11.090.
Lucca, P. S. R.; Nóbrega, L. H. P.; Alves, L. F. A.; Cruz-Silva, C. T. A.; Pacheco, F. P. Rev. Bras. Plant. Med. 2015, 17, 585-591.
Tabanca, N.; Bernier, U. R.; Tsikolia, M.; Becnel, J. J.; Sampson, B.; Werle, C.; Demirci, B.; Başer, K. H.; Blythe, E. K.; Pounders, C.; Wedge, D. E. Nat. Prod. Comm. 2010, 5, 1934578X1000500913.
Taban, A.; Rastegar, S.; Nasirzadeh, M.; Saharkhiz, M. J. Vegetos. 2022, 1-9. DOI: https://doi.org/10.1007/s42535-021-00325-8.
Bahari Meymandi S. A. H.; Alizadeh, O.; Sharafzadeh, S.; Bazrafshan, F.; Amiri, B. Eco-phytochem. J. Med. Plants. 2022, 10, 130-45.
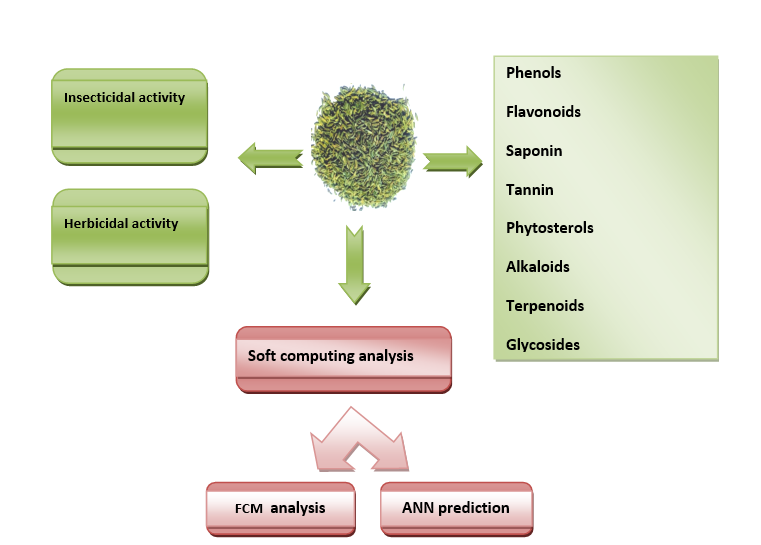
Downloads
Published
Issue
Section
License
Copyright (c) 2025 Nisha Mehra, Garima Tamta, Manish Pant, Neelam Chantola, Viveka Nand

This work is licensed under a Creative Commons Attribution-NonCommercial 4.0 International License.
Authors who publish with this journal agree to the following terms:
- Authors retain copyright and grant the journal right of first publication with the work simultaneously licensed under a Creative Commons Attribution License that allows others to share the work with an acknowledgement of the work's authorship and initial publication in this journal.
- Authors are able to enter into separate, additional contractual arrangements for the non-exclusive distribution of the journal's published version of the work (e.g., post it to an institutional repository or publish it in a book), with an acknowledgement of its initial publication in this journal.
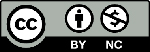